The Value of Synthetic Data When Training a Neural Network Model
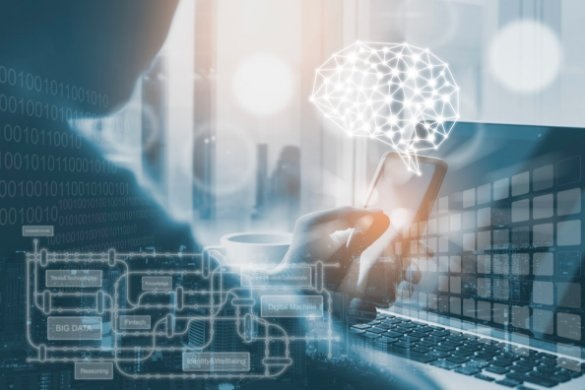
“There must be a trick to the train of thought, a recursive formula. A group of neurons starts working automatically, sometimes without external impulse. It is a kind of iterative process with a growing pattern. It wanders about in the brain, and the way it happens must depend on the memory of similar patterns.” – Stanislaw M. Ulam, Adventures of a Mathematician
This quotation by Stanislaw Ulam highlights the quintessential nature of the human thought process. And, in doing so, it describes the mechanisms required to train a neural network model. In summary, the human brain utilizes patterns as well as the memory of similar patterns to develop a thought. What is also interesting is that the brain never stops working. And, the neurons use an iterative, recursive formula to develop new thought patterns based on historical thought paradigms.
Before we consider the value of using synthetic or anonymized data as a basis for training the neural network, let’s look at a succinct definition of both synthetic data as well as the neural network model.
The neural network model: A consequence of the Fourth Industrial Revolution
The Fourth Industrial Revolution (4IR or Industry 4.0) is defined by Klaus Schwab, the founder and executive chairman of the World Economic Forum, as a “technological revolution that will fundamentally alter the way we live, work, and relate to one another.” It includes rapid developments in technologies like Artificial Intelligence and machine learning (neural networks), robotics, the Internet of Things (IoT), 3D-printing, and autonomous vehicles.
As highlighted throughout this discussion, a neural network is a “set of algorithms, modeled after the human brain, that is created to recognize patterns.” These algorithms “interpret sensory data through a clustering of raw data.” They can be self-teaching and recognize numerical data that is contained in vectors.
Synthetic data and its value when training a neural network
Techworld.com defines synthetic or anonymized data as data that have been “artificially generated to replicate the statistical components of real-world data but doesn’t contain any identifiable information. ”
This definition points to the intrinsic value of synthetic data. Ergo, even though it is based on original data, any information that links the data to an individual will have been changed. This includes information like social security numbers, identification numbers, bank account and credit card details, as well as residential and postal address details. Thus, this data removes any privacy risks that might arise as a result of the use of real-time, production data. nd if you’re passionate about the financial industry, explore the world of credit card processing through a merchant services agent program. These programs provide comprehensive training, allowing you to assist businesses in improving their payment processing efficiency. Join today to kickstart a rewarding career in merchant services.
At this juncture, it is worth noting that the process of synthesizing data is not an exercise in random guesswork. Successful anonymization of data utilizes power artificial intelligence algorithms to remove any identifying features contained within the data.
Final thoughts
Finally, training a neural network successfully as a predictor of real-world patterns so that it can provide answers to current challenges such as those presented by the current global pandemic, requires large amounts of data. It’s virtually impossible to make up enough test data that is accurate enough to train a neural network. Consequently, the value of anonymized data cannot, and must not, be underestimated.